Data Science Upskilling, Improving Data Literacy, and Python’s Rise – Metis Interview

As anyone tangentially involved in the data science and analytics fields is likely aware, there remains a large skills gap between the demand for data talent and professionals who can fill these roles. In addition, for an organization to become data driven and for their data teams to be most effective, there is also a need to build data and analytics skills with professionals across the organization.One of Linda Burtch’s predictions for the data science and analytics market this year was that bootcamps might fill some of demand for companies looking to upskill their current staff, and so we sat down with Mike Galvin, Executive Director of Corporate Training at Metis, a data science and analytics training company. Since Mike leads Metis’ corporate training business, we wanted to get his thoughts on how training programs can address a variety of needs for companies, how Metis addresses both more-technical and less-technical needs, and his thoughts on the future of the upskilling trend.
Burtch Works: Thanks for speaking with us today, Mike! So to jump right in, from our perspective as a recruiting firm, we’ve certainly noticed that hiring professionals with data science and analytics skill sets can be challenging! From our research here we know that a lot of firms are looking to hire quantitative professionals. Have you noticed that companies are becoming more interested in training or “upskilling” current staff as a way to address their hiring needs?
Mike Galvin: That’s a good question and there are several areas where we see interest in training! Firstly, there’s demand for training to “upskill” current data science staff and create career-pathing opportunities to retain talent. Secondly, there’s a need for creating and developing new data scientists. As in, how do you create a learning path to transition interested non-data scientists into data science roles and continually develop and upskill that data science talent. Another area where we see a lot of demand is about better leveraging data science capacity and capability within organizations to derive more value from resources.
Burtch Works: Can you expand a bit on the most common areas you address with training programs?
Mike Galvin: Sure! Our training focuses on a spectrum of non-technical to technical data and analytics skills.One area of focus is on building data literacy for your broader staff. Lessons might cover the language data scientists use, common terminology, what types of problems can be solved, project management workflow, defining the outputs of analyses, and more. This type of instruction enables your broader staff to identify and scope problems within the business and to perform initial analysis without needing the help of a highly technical person. It also improves communication and collaboration between teams.Another focus of our training is working with professionals who might have a somewhat technical background, giving them more tools and techniques they can use. One example would be training analysts in Python so they can automate tasks, work with larger and more complicated datasets, or perform more sophisticated analysis. Another example would be getting them to the point where they can build initial models and proofs of concept to bring to the data science team for troubleshooting and validation.Yet another issue that we address in training is upskilling technical data scientists to manage teams and grow on their career paths. Often this can be in the form of additional technical training beyond raw coding and machine learning skills.
Burtch Works: In terms of the more-technical courses, what do those programs look like?
Mike Galvin: The technical paths typically go in one of two directions, and they’re not completely independent. The first is analytics focused. This goes from basic data analysis like descriptive statistics and data visualization, then progresses on to predictive analytics, machine learning, and more advanced analytical methods. The other track is more tool-based and focuses on things like Python coding skills, Spark, and databases.Depending on the organization, the challenge might revolve around upskilling an existing technical team that is already at the technical end of the data spectrum. In cases like that, it’s more about pushing the team that extra mile or ensuring they’re up-to-date on best-in-class methods.
Burtch Works: Which technical areas would you say are the most in-demand?
Mike Galvin: We match our course offerings to the demand we see in the market. There can sometimes be a misconception that this type of training is always focused on the “latest and greatest” topics. In reality, it’s about upskilling the workforce in the practical skills they need depending on where they are along the data analytics spectrum. Right now we see a lot of demand for Python, Spark, and machine learning, but we cover a range of topics from basic data analysis to more advanced techniques like deep learning, TensorFlow, natural language processing, computer vision, and adversarial networks.
Burtch Works: So you really cover everyone – from the business side and teaching them how to interact with the data team all the way to professionals who are already in highly-technical roles but just want to increase their skill set.
Mike Galvin: That’s correct! We do a lot of training for the not-as-technical folks, along with those who reside in the middle ground. We definitely have more advanced options, but we see a large demand for general data awareness and literacy, as well as training to increase productivity for a staff that’s already using some form of data analytics.
Burtch Works: Since the “biggest demand” is at the not-as-technical side, can you tell us more about what that might look like?
Mike Galvin: Definitely. I would say that there’s a big demand for data literacy, which hits on a number of topics: terminology, use cases, project scoping, lifecycle, and so forth. There’s also a large demand for Python skills to add to a team’s existing toolset or to help them in the transition to using more open source tools. We also have a lot of requests for foundational data analysis and analytics skills up through machine learning.
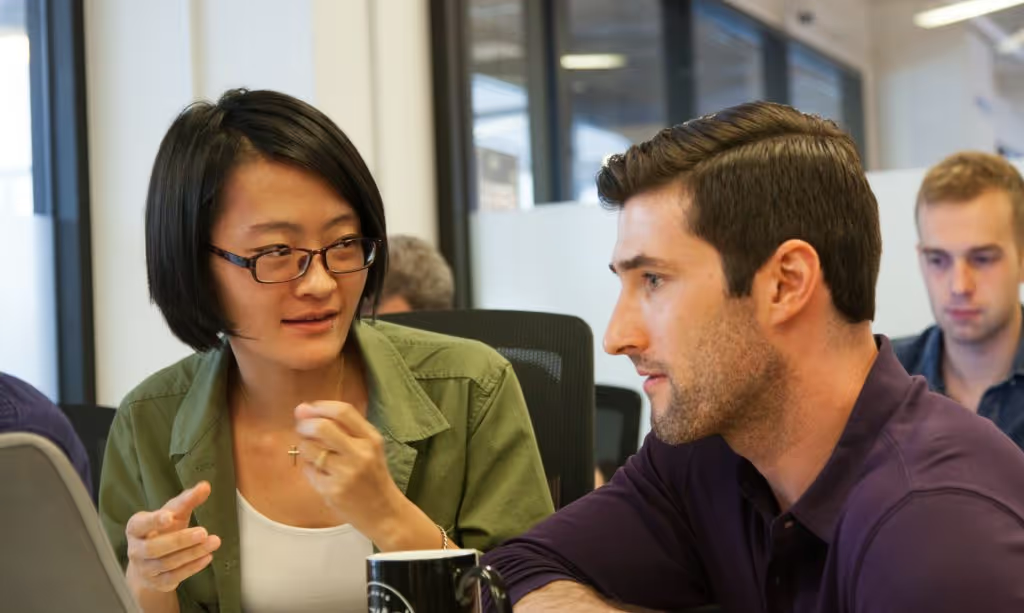
Burtch Works: So you’ve mentioned Python a few times now, are you familiar with our SAS vs. R vs. Python survey that we do? About five years ago we started an annual survey among professionals in the data science and analytics space asking them which tool they prefer, and Python has shown a clear upward trend in recent years. Our prediction is that it kind of takes over as the tool of choice this year. So I’m curious to know if that’s the top tool that you see being used and that people are wanting to learn? Or are there other tools that people are using?
Mike Galvin: I would agree with what you found! It seems that most people use one of those three: SAS, R, or Python. Metis is primarily a Python shop, but even with that being said, just from the conversations I’ve had in the industry, Python’s growth is the trend.We get some requests for R, but not a lot, and it’s by far overshadowed by the amount of Python requests we receive. We actually do a lot of trainings with SAS users transitioning to Python.
Burtch Works: So are your programs set courses or are they customizable depending on each client? Depending on what they’re looking for and what problems they’re trying to address?
Mike Galvin: Both. We have a broad portfolio of offerings, which includes off-the-shelf courses and custom programs with various modality options (in-person, live online, a hybrid, etc). Our off-the-shelf offerings allow us to pick courses with outcomes that align with business. And then, depending on the scope and scale of the program and the needs of the organization, there are varying levels of customization that we can include to contextualize what we already have to fit your needs. Customized programs can include integrating distinct tools, projects, and use cases into the program and spacing the training out with mixed modalities to improve retention.
Burtch Works: So could you walk us through a typical setup for a program that you might run for a client? How are they structured in terms of class time or length, class size, and do they get out-of-class work?
Mike Galvin: It’s hard to generalize because this varies depending on the topic, audience, and delivery mode. We always keep in mind the outcomes and focus on answering the question: what do people need to be able to do within their organization after this training? Then we move on to think about the time frame you’re working with, who is the audience, and how do we teach them and get it to stick.For a one-time training that’s delivered in-person, the class size is typically 12-30 people. Classes are a mixture of lectures touching on conceptual topics and practical issues, practical exercises and projects, and other activities like case studies, small group work, and group discussions.
Burtch Works: Would you say you work more with off-the-shelf programs or custom programs?
Mike Galvin: We do a mix of both, but I would say it normally starts with off-the-shelf products, and we help guide the client to which ones are going to help them solve their particular problems. We might do one or two engagements, starting with something off-the-shelf with some contextualization as a pilot, and then we’d go from there depending on the scale of what they’re looking for. At that point, we start getting into the more customizable programs.
Burtch Works: What types of companies are you working with? Are there particular industries that are more invested?
Mike Galvin: It’s really across the board as we work with companies of all sizes, sectors, and industries. We’ve done everything from smaller startups to Fortune 100 companies, but we’ve worked most extensively with mid-cap to Fortune 100 clients. As far as sector goes, we’ve worked with Financial Services, Manufacturing, Industrial, Oil & Gas, Technology, Retail, CPG, and Management Consulting. Sometimes the problems vary depending on the industry, but there are a lot of commonalities, and the need for upskilling in analytics is there across the board.
Burtch Works: It seems like for companies, if they don’t have the resources or knowledge-base to create their own huge, internal training programs, that services like yours would be a pretty good solution. If we could ask you to look into the future and make some predictions, do you have any thoughts on how the upskilling and training trend will play out in this space?
Mike Galvin: It’s definitely going to continue to increase. We hear a lot about the demand for data scientists and difficulties with talent acquisition and retention. If you think about the existing workforce, data and analytics skills are either critical already, or they will be in the near future within all organizations regardless of size, geography, and sector. As the importance of data science and analytics in organizations increases, learning and development programs will only become more important.A common pitfall I see is companies trying to do this sort of training internally. This pulls specialists away from their regular work and can become problematic as they try to balance the two. It’s also important to have an education partner who knows how to design, develop, and teach these types of programs.In terms of how it all plays out in the long run, that remains to be seen. I expect to see continuous innovations in education around delivery methods, user interaction, and assessments.
Want to learn more?
Reach out to Mike if you'd like to discuss upskilling all levels of your workforce in data and analytics.